How did you go bankrupt?
Hemingway, The Sun Also Rises
Two ways. Gradually, then suddenly.
The late Gordon Moore famously observed that computing power doubles every two years. For a long time you’d be forgiven for barely noticing. Our devices got faster and smaller, sure, but ultimately they were still computers doing computer things, just more efficiently.
But as Hemingway describes, exponential curves start slow then get quick. Suddenly we’re seeing astonishing change. Powerful cloud computing, paired with new programming techniques and oceans of data, is writing convincing essays, passing exams, and painting compelling pictures on request. Bill Gates says these generative AI systems are the most important technology advance in decades. He’s right, and progress is likely to accelerate from here. But as AI systems mature they also pose deep questions about the future we want to inhabit, and who gets to build it.
Bias
Modern AI systems consume vast quantities of data, building predictive models that then work with new data sets. Say you’re building an AI that predicts whether a criminal might reoffend. To train your system you’ll feed it data on previous arrests, sentences, and reoffending rates, and ask it to find patterns. It will probably find these patterns with ease, but not for the reasons we might hope. Data always describes the past, and the past is biased.
Thanks to systemic racism in the police and the courts, our algorithm will learn that people from certain communities have historically been arrested more often and sentenced more heavily. It’s likely, then, our AI will suggest we continue this pattern. A system that appears neutral – just numbers and code – will itself make biased recommendations. After all, that’s all it was ever taught.
This is precisely what happened with COMPAS, a criminal risk system used across the US. An exposé by ProPublica argued the software was biased against Black defendants, predicting they’d be more likely to commit future crimes than other groups.
The findings are still contested, but COMPAS is no isolated case. When MIT researcher Joy Buolamwini found facial recognition systems didn’t recognise her dark skin, she fabricated a white mask and wore that instead. The system then recognised her easily. In 2015, Amazon scrapped a recruitment algorithm that marked candidates down for attending all-women’s colleges. In both cases, these systems were just reflecting the skewed training data they’d been fed. The facial recognition system had learned to recognise mostly white people; the hiring system discovered that Amazon had historically overlooked women for technical roles.
Learning from these problems, technologists are working hard to reduce bias from training data and machine-learning algorithms. But this turns out to be difficult. There are plenty of statistical methods, but the biggest challenge is philosophical: you have to define what fairness means. Make a system fairer by one definition and you often create unfairness in another direction. So tackling bias often means choosing which biases to erase and which to accept: a complex, human, and social decision that computers aren’t well suited to answer.
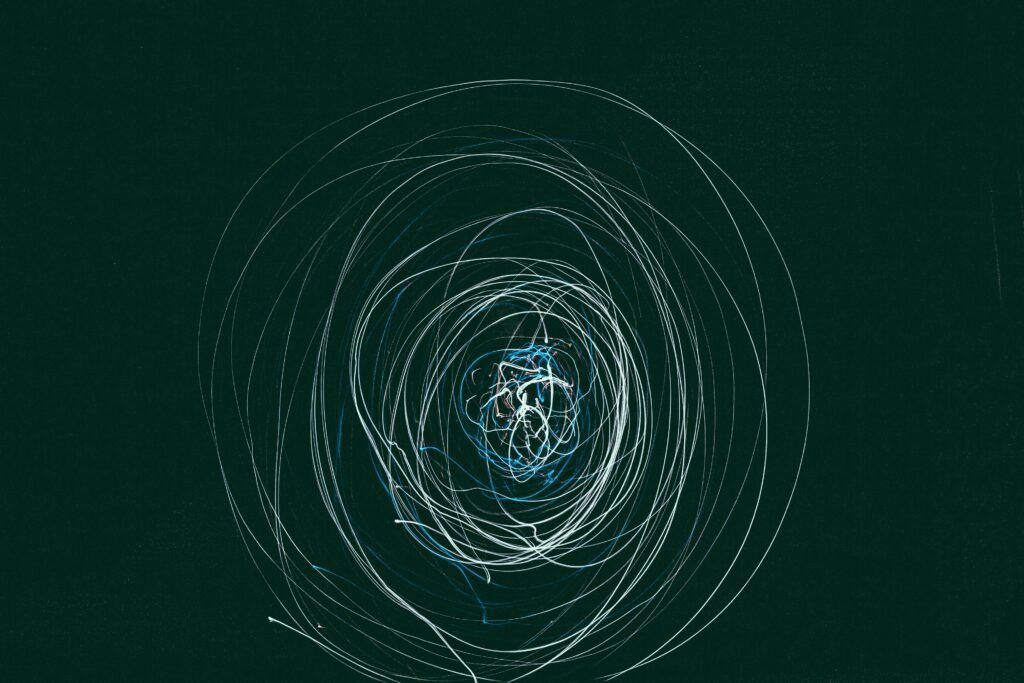
Explaining AI decisions
Even if our AI is fair, can it explain itself?
Modern AI systems don’t ‘think’ in the way we do, so they struggle to describe their decisions in logical terms humans can understand. The best most systems can offer for now is a series of numbers (weights of nodes in a neural network), which are meaningless in isolation.
Yet AI is influencing more and more critical human decisions, such as allocating medical resources, or approving or denying people loans and jobs. With impacts this serious, it’s right that we should expect these systems to explain their decisions. Until that time, there’s a fair case to argue AIs shouldn’t be used when lives and livelihoods are on the line.
Worse, some AI outputs are simply wrong. New language models like ChatGPT are famous for ‘hallucinating’: a cute euphemism for what is really happening, which is outright fabrication of information. Ask ChatGPT to give references for, say, a scientific theory and it will conjure up academic references with plausible titles and authors. But your local librarian will never be able to find these papers, because they simply don‘t exist.
Whether consciously or not, AI manufacturers have decided to prioritise plausibility over accuracy. It means AI systems are impressive, but in a world plagued by conspiracy and disinformation this decision only deepens the problem.
Privacy
Technology has long moulded the history – and even the idea itself – of privacy. The advent of photography prompted the first US laws, and in recent years online tracking has further influenced the debate. No surprise then that regulators have set up new privacy protections, but even these could be undone by powerful AI.
Data is often anonymised to protect privacy, but this is less effective than we might hope. Privacy specialists have been able to identify individuals from anonymised records with worrying ease. In one case, two researchers combined Netflix and IMDb data to identify users and deduce their political leanings. In another, Carnegie Mellon professor Latanya Sweeney found she could identify more than half of the US population using only public data on gender, date of birth, and town of residence.
A future AI armed with the right data could be even better at identifying individuals than these skilled researchers. Even CCTV footage could be retrospectively analysed and mapped to a facial recognition database, building a map of your historical movements and a list of people you spend time with. Data that is harmless today may make you traceable tomorrow.
Overseeing the future of AI
Beyond the contemporary issues lurk deeper long-term questions. What should and shouldn’t we automate? Should we create lethal autonomous weapons, or should a human always take charge of life-and-death decisions? How will we earn a living and even find meaning if AIs eliminate jobs? And if we ever create AIs that are smarter than us, will they value the same things we value – not least human life itself?
These are profound questions which deserve democratic debate. But today only a tiny cluster of AI firms, often funded by the world’s richest and most powerful people, are calling the shots. Is this the future we want?
I make a living helping technologists understand the social, political, and ethical impacts of AI and other emerging technologies. In recent years I’ve seen some progress, although the field of technology ethics is still nascent. What we really need now is outside help.
One answer is to regulate AI. This is starting to happen – particularly in the EU – but progress is modest. Politicians see AI as an economic growth lever and are worried about hampering its development. Even then, regulation is never enough on its own. The severity and urgency of the questions AIs poses means we also need a wider public discussion.
So now is the time to pay attention to what’s happening with AI and to ask questions. Ask how it is used in your public spaces, your schools, your workplaces, by your police, by your governments, by your militaries. Urge those in power to justify their decisions and discuss the safeguards they have put in place to ensure AI’s effects are positive. The issues involved are too important to be left to technologists.
Leave a Reply